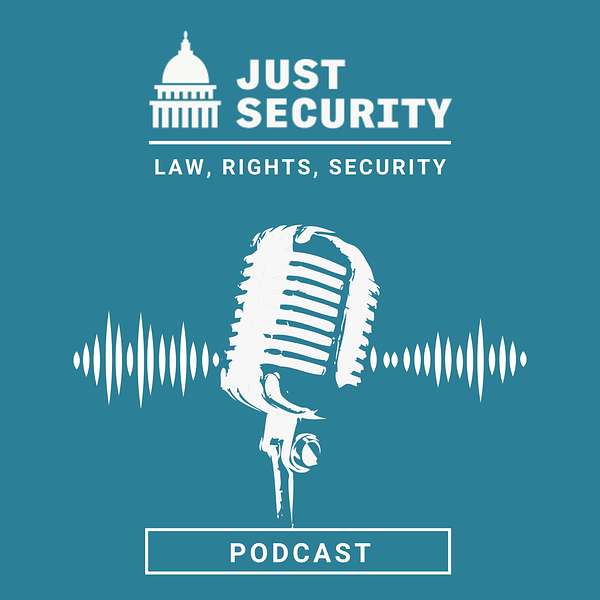
The Just Security Podcast
The Just Security Podcast
Diving Deeper into DeepSeek
The tech industry is calling this AI’s “Sputnik Moment” – and President Donald Trump has said it’s a “wake-up call” for U.S. companies. We’re talking about DeepSeek, the Chinese AI startup that has rapidly emerged as a formidable contender in the global AI race.
DeepSeek is making waves for developing powerful open-source language models that rival leading U.S. competitors – at a fraction of the cost and with far lower computational requirements.
The DeepSeek saga raises urgent questions about China’s AI ambitions, the future of U.S. technological leadership, and the strategic implications of open-source AI models. How did DeepSeek get here? What does its rise mean for competition between China and the United States? And how should U.S. policymakers respond?
Today, we’re going beyond the headlines to dive deeper into DeepSeek. We’ll explore popular myths and misconceptions surrounding DeepSeek, the technology behind it, and what it means for national security and U.S. policy going forward. Joining the show to unpack these developments are leading experts in the field: Dr. Keegan McBride, Lauren Wagner, and Lennart Heim
Keegan is a Lecturer at the University of Oxford and an Adjunct Senior Fellow at the Center for a New American Security. Lauren is a researcher and investor, now with ARC Prize, previously worked at Meta and Google. And Lennart is a researcher at RAND and a professor of policy analysis at the Pardee RAND Graduate School.
This episode was hosted by Dr. Brianna Rosen, Director of Just Security’s AI and Emerging Technologies Initiative and Senior Research Associate at the University of Oxford.
Show Notes:
- Lennart Heim (LinkedIn – Website – X)
- Keegan McBride (LinkedIn – X)
- Brianna Rosen (LinkedIn – X – Bluesky)
- Lauren Wagner (LinkedIn — X)
- Lennart’s Just Security article with Konstantin F. Pilz (Bluesky – LinkedIn – Website – X) “What DeepSeek Really Changes About AI Competition”
- Keegan’s Just Security article “Open Source AI: The Overlooked National Security Imperative”
- Just Security’s Artificial Intelligence coverage
- Just Security’s Tech Policy under Trump 2.0 Series
- Music: “Broken” by David Bullard from Uppbeat: https://uppbeat.io/t/david-bullard/broken (License code: OSC7K3LCPSGXISVI)
Brianna Rosen: The tech industry is calling this AI’s “Sputnik moment” — and President Trump has said it’s a “wake-up call” for U.S. companies. Like everyone else, we’re talking about DeepSeek, the Chinese AI startup that has rapidly emerged as a formidable contender in the global AI race.
DeepSeek has been making waves for developing powerful open-source language models that rival leading U.S. competitors — at a fraction of the cost and with far lower computational requirements. The company recently released DeepSeek-R1, a model reported to be 20 to 50 times less expensive than OpenAI’s o1 model that is comparable in performance. Meanwhile, DeepSeek’s AI assistant, powered by its V3 model, has overtaken ChatGPT to become the most popular free application on Apple’s U.S. App Store.
The DeepSeek saga raises urgent questions about China’s AI ambitions, the future of U.S. technological leadership, and the strategic implications of open-source AI models. How did DeepSeek get here? What does its rise mean for competition between China and the United States? And how should U.S. policymakers respond?
This is the Just Security Podcast. I’m Dr. Brianna Rosen, Director of Just Security’s AI and Emerging Technologies Initiative and Senior Research Associate at the University of Oxford.
Today, we’re going beyond the headlines to dive deeper into DeepSeek. We’ll explore popular myths and misconceptions surrounding DeepSeek, the technology behind it, and what it means for national security and U.S. policy going forward. Joining me today to unpack these developments are leading experts in the field: Dr. Keegan McBride, lecturer at the University of Oxford and an Adjunct Senior Fellow at the Center for a New American Security; Lauren Wagner, researcher and investor, now with ARC Prize, previously worked at Meta and Google; and Lennart Heim, researcher at RAND and a professor of policy analysis at the Pardee RAND Graduate School.
Keegan, Lauren and Lennart, welcome to the show. Keegan, we're going to delve deeper into DeepSeek in a minute, but I want to step back and put this all into context. So, what does it really mean to lead in AI and why does this matter? Walk us through why this is such a critical moment in tech policy.
Keegan McBride: I think if we look back at history, we saw how transformative the internet was as one example, and basically, it set the foundation for today's digital economy. And countries that were able to take advantage of building out the infrastructure for the internet early on have huge advantages today.
My guess, and I think many out there will sort of agree with this, is that AI has the same potential. It's going to be completely transformative, impact our economies, national security, science, manufacturing. And realistically, there are two countries at the moment who have the capabilities to sort of lead at the frontier of AI development. That's the United States and China, which are two countries that have also been increasingly, let's say, hesitant to engage with one another, rising tensions, you know, growth in fears of conflict over Taiwan, amongst other things. And so given the fact that this technology is so transformative, and it has such a potential to sort of impact the broader geopolitical distribution of power, there's huge demand to be the country that leads and drives its development, and that's really what sort of sets the foundation for a lot of this stuff.
Brianna: So, let's dig deeper into DeepSeek itself and what we know and don't know about it. Now, there's been a lot of debate about the rise of DeepSeek and with the release these new models. Lauren, I want to turn to you to unpack what are some of the key myths and misconceptions surrounding this debate? What explains DeepSeek’s efficiency gain? Is this really genuine innovation, or is it just following predictable trends aligned with what we would expect to see with industry trends?
Lauren Wagner: I think it's a little bit of both. And I don't like looking at these questions and providing a black or white answer. There's really a lot of nuance here. And what I like to do first is really start at the company and look at the company. So, I've worked at startups, I've worked at Google, I've worked at Meta. I'm like, what is the culture of a company that would yield potential innovations or efficiency gains and things like this? So, what we know about DeepSeek, it’s about 150 people. They recruit only from people and researchers in China, and they really focus on collaboration and creativity. Those are their primary values as an organization, and they provide essentially almost unlimited compute to their employees and pay them quite well. And so, it's kind of a breeding ground for new ideas and experimentation in AI, and I think that might lead to some of the gains that we're seeing, especially in comparison to some of the bigger companies here.
So, when we talk about efficiency gains, there has been a curve and a trend line that we've been following now for quite a long period, at least in AI terms, where you could use less compute to achieve specific gains in capability. And so, what we're seeing here kind of continues along those lines, where we're seeing less of an emphasis on using compute for training and more of an emphasis on inference. And when you focus on inference, that actually leads to models and outputs that are more reliable, that are more trustworthy, and so, DeepSeek is definitely capitalizing on that or, you know, advancing and building on that trend. But I do think that there — and we know that there are special things about DeepSeek that have resulted in algorithmic advancements and other innovations that have led them to be able to achieve greater things than other major labs, especially those in the U.S.
Brianna: I want to get more into the compute versus inference debate in a minute, but first, I want to turn to you, Lennart, to unpack a little bit more how these latest models compare to the leading U.S. models. Basically, how worried should U.S. policy makers be about these developments, and how can we really compare training costs across companies when the leading U.S. companies are not publicly releasing this information? Can we really compare the performance and the training costs, etc., with DeepSeek?
Lennart Heim: Yeah, I think the comparison is a fantastic point. As Lauren was saying, this is a genuine thing, like, there’s, like, general innovation happening here, but on your side, we just really have a hard time comparing to what OpenAI, Meta and all of these other companies are doing, right? We don't know what their latest training costs are. We don't even know the size of these models. All of this is like, behind closed doors. And I think this is a trend we've been basically, I would say, basically, after GPT-3, they stopped publishing and announcing how exactly these models work, what the underlying architecture is, how much it costs. We don't even know the size of the clusters. Again, we don't really know for DeepSeek as well, but it's like, a bunch of speculation around it. But we're like, we're really in the dark on all of these kinds of things.
I think part of the reason why we got so much attention on DeepSeek is, they were one of the rare companies who put out the training cost in their V3 paper, right? Before — I've been doing this now for a couple of years— we always try to first estimate the training compute on various different methods based on what we know, and then we try to put cost of it, whereas, like, DeepSeek actually put a number on it and use the method there, and which then, unfortunately, many people got wrong, because it's only the cost for the final pre-training ground. There's like, way more, right? Like, buying the hardware costs you way more. You still need to pay your researchers. You have a bunch of experiments, and I think this is like sometimes not being paid enough attention to.
And then on your point regarding performance, we had DeepSeek Version 3, which was, like this big pre trained model, which was better than GPT-4. And I think roughly on 4o performance, which is impressive. I think that's why it caught attention. But I think we saw the most attention just two weeks ago with DeepSeek R1, which is basically on pair with OpenAI's o1 model, which I think OpenAI first announced in September and then made publicly available in December. And that's impressive performance. It's a leading reasoning model.
On the other side, OpenAI hasn't been sleeping, right? They announced at the end of December, and I think just, also just the other day, o3, right? The next version, the next iteration of this model there. And this is generally what I expect. Now here, we’re this new paradigm, which is test time, compute paradigms, inference paradigm. But again, it doesn't mean we leave the whole paradigm behind. We probably will continue innovating at both at the same time to just continue keeping the attributes going up. But in this new paradigm, I expect we will see, like, more improvements quickly, because everybody's not focusing on. We now even see, again, researchers from academic institutions also paying more attention to this, because this is a fairly computer-efficient paradigm, right? With like, new ideas there, I expect they're more low hanging fruits. And then generally, I expect this year we will see more innovation there with DeepSeek, but also all of the other companies, again, getting, gaining more capabilities there for relatively little compute.
Brianna Rosen: So, DeepSeek did this on a relatively small compute budget vis-à-vis leading U.S. companies and Lennart, you wrote for Just Security this morning that the narrative that DeepSeek diminishes the importance of compute is misleading. Can you walk us through your logic behind that a little bit? So, like, what does the R1 paper say about distillation, and what does this mean for the future of inference versus compute? And then I also want to bring Lauren into the discussion as well.
Lennart: Yeah, so, Lauren was talking about it. We see efficiency trends for the last decade in computing. This is nothing new. We actually saw it over the last 100 years in computing. Moore's law being like the most famous example of this. Turns out there's an exponential going up and down, depending on what you put on the Y axis. Things are getting more efficient over time, and machine learning has not been an exception there. And I was personally surprised how much it caught ours by surprise. I was like, yeah, this is like, within the trends line, trend lines roughly, and again, maybe it just goes back to, we didn't have a real comparison class here, and that's why many people then came to the conclusion, well, compute’s not important anymore. Well, binaries are usually hard. Of course, it's still important. It's just less important to achieve a given capability over time. And that's the trend we've always seen.
If you now want to have an AI model, which is as good as the best model on, for example, ImageNet, a famous benchmark where you try to classify pictures, that's significantly cheaper, because it has 1000 bucks, now costs us a couple of cents to achieve this performance. But it doesn't mean we're good with being good at ImageNet, right? We continue pushing forward the frontier. Are we now going to stop and say, oh, one is enough to transform the economy? I don't think so, right? So, we always have, like, what we call this access effect — people getting access to the same capabilities for less compute, for less compute over time, but the other side, with a performance effect — you get better performance for the same budget. And this is what we will continue seeing. People will continue scaling these models, getting better and better capabilities, again, to then drive this economic, valuable task going forward.
So, we have both of these trends happening at the same time, and I don't think this then fundamentally challenges computing resources being important. It challenges them for achieving certain capability being important. But again, we want to push these capabilities forward. And then compute is important for more than just training, and these models compute, we need this for experimentation, right? There have been these rumors of DeepSeek having 50,000 hoppers having, like, a lot of computing resources. DeepSeek was actually one of the first labs in Asia having access to 10,000 A100s before any export controls. They were early on the game. They were early betting on compute, right? And even if you look at their own quotes, that being compute is like the key here, it's just wrong to only think about compute being this one thing which you need for training the model. There's like this more purposeful use for just like experimenting and eventually, also deploying these AI capabilities here, right? It's beautiful to have a good model and have really great benchmarks. Eventually, you want to make money out of it and drive economic change. And whatever you're trying to achieve here, this means you need to deploy these models. And what we saw with DeepSeek, shortly after release, at some point, they stopped everyone who didn't have a Chinese phone number from signing up, right? I would read into this, well, looks like they had a little bit of a little bit of a compute crunch there. They didn't have enough resources to deploy it. But it's kind of bad, because that's how they make money, right? That's how compute is being used as this is how you drive economic change. So, this is, like, another purpose, like, use of compute, just like, it's really important to understand. And again, just this naive take of like, exponents don't work anymore. I think, like, really, this is the forest for the trees here.
Brianna: Lauren, do you agree with Lennart's characterization that this actually makes compute more important, not less? And how should we think about this in the context of Trump's policy moves with the $500 billion Stargate AI infrastructure project and all of this going on in the U.S.?
Lauren: Yeah, so last week was really interesting because I spoke to a lot of different journalists, and I actually spent a good amount of time at the Council on Foreign Relations, so I was able to get a lot of different kinds of feedback around what was going on and help calibrate my own point of view. So, I agree with everything that Lennart said, and then I also think about what folks said to me last week. So, the question about Stargate was something that journalists brought to me multiple times — is Stargate now a project that we don't need and something that we shouldn't invest in? And so, I think people, some people, their first reaction is essentially, why are we investing all of this money into data centers and compute when it seems like we don't need it? And hearing Lennart's, you know, explanation, I think that that's definitely not the case, especially when you have models in the hands of more people if they are cheaper to use and cheaper to run, and they actually end up being more reliable and trustworthy. That's something the industry is still struggling with. So, any advancements on that front, I welcome, and I'm very excited about.
To the point about export controls, that is something else that also stoked a lot of fear among people who think about the geopolitical landscape day to day. And so, this question of, are the measures that the U.S. government is putting in place, are those measures adequate? Are they working? I think what we're finding out day by day, there's kind of a leaking out of information. I feel like every day I'm learning more and more and getting a different, slightly different perspective on what's happening now. There was an article in last night or today about how Nvidia has seen these export controls, and they've been able to kind of out innovate and provide other types of products to China that may not be subject to those export controls. So, I think we're in this period where we want to have concrete answers about what's going on, and it seems that we're trying to triangulate data and get a better sense of what's happening on the ground in China and how what the U.S. does, and policy from the U.S. perspective, how that impacts China and the rest of the world. And so, it's important to keep on top of these things that have folks who can synthesize the information in real time, so that we get an accurate perspective. And there isn't this gut reaction to say, to have this kind of binary black and white thinking, it's all good, it's all bad.
Keegan: Can I just sort of build off of some of what we're talking about here? I think it's really important to clarify that, yes, export controls are in place, but that doesn't necessarily mean they were working as intended. And I think there's quite a common mistake that people make where they hear that, okay, there was export controls in place. This means that China couldn't obtain, sort of, state-of-the-art GPUs, but for a pretty significant period of time, that just wasn't true. Yes, there were export controls on the book, there were exceptions, and Nvidia was making sort of new products to get around them. As Lauren was just saying, it was easy to smuggle them in.
Brianna: And Keegan, the Biden administration only closed some of those loopholes that you mentioned. They only closed them in October 2023, right? So, it's been, like, over, a little bit over a year since we've had stronger export controls in place. Is that even really enough time for them to take effect in a meaningful way?
Keegan: I mean, yes, they were in place, but I think what you saw is the government was probably six months to a year behind in terms of updating export controls to be with the situation on the ground actually was, and the implications of this, basically, are for the foreseeable future, at least for the next couple of years. I think it's fair to say that China, from a compute standpoint, is not necessarily as far behind as the U.S. government perhaps would have wanted. But what that doesn't mean is that that's going to remain the status quo for the next 5,10, 15 years. I think what you're going to see in the new sort of administration is a big crackdown on export controls, taking enforcement a bit more seriously, and pushing some of these a little bit further.
So, that might mean in the next one year, two years, we continue to see advancements out of China, but they're absolutely going to struggle to bring their models to the world. They're going to struggle to diffuse their solutions as they try to scale them, as we've been talking about earlier. Yes, they might be able to create and sort of innovate domestically, that will be okay for their economy, but the idea that in the face of increasingly strict export controls that are properly applied, properly enforced, with growing, sort of, agreement amongst the U.S. and its allies that China being a world leader in AI, you know, is a bad thing. I think it's, I'm not going to say it's a one-off, but the future situation is going to be quite a bit different than it currently is, particularly as U.S. innovation continues to speed ahead, which it’s actually going to, you know, to do.
Lennart: On top of Keegan’s points. I think just like everybody who's been following export controls knows they're not perfect, right? And I love that the world sometimes thinks policy just works like perfectly. You put it out there, and then you got it right. I don't think this will ever be the case for AI governance or AI policy in general, which is, like, a really fast-moving target, and regulating it well, it's like, surprisingly hard. So, I think people should actually have more of a prior that we don't always get AI regulation right. Unfortunately, people are trying their best, and again, the initial export controls were early, remember October 2022, this is even before ChatGPT. So, credit to the Biden administration back then, even credit to the Trump administration to identifying semiconductors as a key target for like, like, tech competition, right? Because eventually Trump and others did ban Huawei and put other companies on the entity list, and did ban extreme ultraviolet lithography, which are the cutting edge machines you need to put AI chips on going to China.
So, they were early on this. Then these export controls came out trying to target specific chips. Unfortunately, they got some numbers wrong there. As Keegan was saying, we basically had a year where they could just buy these workarounds and Nvidia just, you know, again, they follow the law completely right. But they also knew that these chips are as good, and they just thought they can take an opportunity of this, and then it just took a year to fix it. This just highlights the needs of like, it would be great if the Bureau for Industry and Security, the Department of Commerce and everybody else in the agency process could act quicker on these kinds of things. A year within AI is making a big difference, right? And this is just what we've been seeing there.
And then on the other point, what does this mean when they only buy it in the future, again, like pre-empting here, what's going to happen? They will continue developing good models. For people to just expect right now, oh yeah, they didn't hit yet, because it's only one year in, but two years in we got it, recovered. Won't happen. It's like, won't be this binary, right? Training a competitive model will always be easier than, regarding compute constraints, than training 10 competitive models, deploying it to billions of users. So, it's, like, really important to understand where do these export controls eventually hit? And I think it's best to think about, they have limited access to a resource. When you have limited access to resources, you spend it differently. So, they could be saying, we only train one good model and deploy it to 10 million users, whereas the U.S. and the rest of the world could say, well, actually, we got five competitive AI companies, and we can deploy to billions of users at the same time.
This is not what you see when you only compare to benchmark-to-benchmark comparison, right? So, this comparison will always be the hardest. And my last point, connecting to just like recent developments that people are listening, they might have seen OpenAI just releasing something which is called deep research. And I think that's like a nice point to, like, really understand why computer is important. Deep research is just a product where you basically tell an OpenAI agent built on o3, so the most recent reasoning model, you ask a question, and it goes off. It's like a research assistant that does some research there. When this model goes off, it's running on computation resources. It's reading the internet. It's reading a bunch of documents. It's processing them. It's thinking through them. All of this requires compute, and just only highlights the importance of compute there.
So going back, they might have one model, but they are one model. If they send it off to do research, it can read less documents. It cannot think for that long, and it can serve less users. This is more of an impact you will be seeing in the future. And again, this matters a lot for transformative impact that, like, the energy, which I love, sometimes like to use. It literally determines how many AI workers you have. How many workers you have is a key function of your economy here. So, we should expect export controls to hit harder there on these kinds of impacts. Whereas, if it's about like a single use case, or like a single company using AI to accelerate their research process, again, compute expert controls will be having a harder time biting here. So, it’s just, like, really critical to understand, like, where does it bite? Where does it not bite? And I think just many people have, like, this really simplistic and, like, optimistic view that will just lead to this binary — out of the game, we got it. Unfortunately, this is not true.
Brianna: Thinking about the future of export controls and the lessons learned from DeepSeek, it strikes me that one of the few Biden era legacies that the Trump administration has not yet overturned is the AI diffusion framework passed in the last week before the inauguration. And the framework, among other things, sought to limit chip diversion to countries like China and Russia, as well as quite a few handful of countries in that second restricted tier in the Middle East, India and even parts of the EU. And this was really a concentrated bipartisan effort to control the diffusion of AI technology worldwide.
So, when we think about DeepSeek and some of the lessons learned from this going forward, do you think the AI diffusion framework, as it currently stands, is sufficient for strengthening export controls? Or does the Trump administration need to do more to build upon this framework?
Lennart: Yeah, happy to take this one. Export controls have been a bipartisan issue. U.S. tech competition on semiconductors and AI has been a bipartisan issue, and we just had Lutnick testifying during his hearings. He likes export controls, and he's in favor of them, and I applaud, and I think that's the right move here.
We talked about problems of export controls. The diffusion framework is trying to solve a bunch of existing problems, right? We know chip smuggling is a thing. We know Chinese entities are just going to Malaysia and build data centers there. The diffusion framework is basically trying to solve this. So, the way, how would I phrase it, is, we got real issues with export controls. The diffusion framework is one way of solving it by making sure that the diversion for other countries is, like, more limited. And again, the diffusion framework is not only about solving the problem of China and the PRC. It's also about, like, solving a problem with a bunch of countries in the Middle East, where I think people should also be a bit more careful of how much access to compute they eventually get to.
But, we should not be mistaken again here that it solves all of the problems. The diffusion framework builds on top of existing export controls, right? This means it uses the same export control classification number as the previous ones. This means the AI chips and controls are still the same. So, we just had in October 2023, we patched export controls. We're now covering the H100. But of course, what did Nvidia do? They developed a new chip, right? This is the so-called H20 which people are now chatting about. And this chip is definitely worse for workloads which mostly rely on computational performance. So the so-called flops, the floating point operations per second, which is mostly the training one, but it's actually a real competitive chip, if you think about workloads which mostly require a bunch of memory access, a bunch of read and writing. This is the case because they have access to this high bandwidth memory.
I don't get lost into the technical details here. But there's still a chip out there. It's definitely not the best chip out there available, but it's good for some workloads, and it's been seeing, according to reporting, up to a million units per year being sold to the PRC and more. And I think this chip is getting more and more attention because, turns out, training is important, but actually this deployment compute, this inference, also gets the capabilities, right? And I think this changes, like the math on this, but you also think you want to cover a wider range of chips, because they now also lead to impact and again, and you also want to have a broader impact on the deployment here. So, I think people should pay really careful attention to this chip. And again, if you follow the AI developments, the chip just became more important, and the move here might eventually then also be restricting it. And notably, in the most recent export controls update on December 3, we already saw the Biden demonstration restricting high bandwidth memory units from going to China. So, the PRC is currently not allowed to equip their own AI chips with HBM, with high bandwidth memory, but they're allowed to buy chips of high bandwidth memory, which are really competitive here. So, you could just think this is just like a logical consequence eventually to also trying to restrict this chip.
Brianna: Does anyone else want to jump in on the export control debate?
Keegan: I think that there are two things that we need to sort out here. One is the actual effectiveness of the sort of AI diffusion framework that was put in place. Does it represent an improvement on processes that were in place prior to it? I think yeah, in many ways. Is the communication and the discussion around it a problem? Probably, yeah. And I think this is, you know, a really important point to bring out, which is that, for the past couple of years, to be honest, a lot of the messaging around AI, AI development has maybe not been the best, where there was this idea that AI could be sort of locked down to a couple of companies, that open source was dangerous, that, you know, by trusting a handful of companies, we would somehow come up on top, sort of, over China.
And what that has done, even if that wasn't sort of put in place like as a policy per se, it's just how it's talked about, it has a chilling effect on innovation. We saw companies actually sort of not engaging with particular partners because they were scared to, or they thought new regulations might be on the way. It wasn't clear what the future for open source might be. On the flip side, China didn't have these same concerns, per se, and was innovating and innovating and innovating. The U.S. was as well. But with the diffusion framework, we now have a whole bunch of allies, for example, in NATO, who have been classified as tier two countries. On some ways, they do actually still have access to compute. They still can access, you know, sort of cutting-edge AI models. On the other hand, they've all been told that they're basically second-class countries, and while, you know, in practice, they're still okay when it comes to the access of AI, to the access to compute, it's the messaging. And this is where I start to get a little bit concerned, because the real value from AI is going to be having at scale, sort of globally, having different countries engaging with American AI-based solutions, you know, having their economies working with American AI-based solutions. That's much harder to do when they don't think they can trust the U.S.
And what I have seen a lot of, particularly from Eastern European countries who have sort of been lumped into the tier two definition, is that they will say something to the effect of, this means that we should start looking towards open source AI, and at the moment, China has the best solutions on the market. The U.S. has Llama. My guess is that we're going to start seeing changes to this sort of ecosystem. But I would just really encourage people to not underestimate the importance that messaging and tone and communication has on some of these things, because there's a big disconnect between the policies, which I think in many ways, are actually quite good, versus how they're talked about, which is sending a message that this is only for us, and you can't have it and you don't want to trust us. And whether or not that's what's intended, this is certainly how it's being perceived by certain allies.
Brianna: Yeah, and of course, this is an open source model. DeepSeek released an open source model, which means anyone can download and modify it. And Keegan, you had written for Just Security in June that the failure to foster and sustain open source AI innovation would really have disastrous consequences for the West in national security terms, because essentially, it’d allow Chinese firms to develop all of these open-source AI models, like the DeepSeek model, which could form the foundation of the world's critical infrastructure, then thereby also embedding techno-authoritarian values into that infrastructure, rather than Western norms and values. So, just walk us through a little bit, what are the national security implications of these developments? How does the open source nature of these models affect global AI development, and what does that mean in terms of, you know, what U.S. policymakers should do about it? I want to bring Lennart into this debate as well on the open source versus closed source models.
Keegan: I mean, I think what we have seen is that there's growing competition between, let's say, the more liberal and democratically aligned West and a more technical, authoritarian aligned alliance, like, including Russia and China. Both sides are sort of angling to set the rules and the foundations for what the future of our digital world is going to look like. We already saw a lot of this, sort of, reflecting back to my earlier answer with the internet, we still see a lot of this playing out today, with, you know, countries actively working to bring about new authoritarian aligned norms to the sort of governance of the internet.
The fact of the matter is, AI is going to be incredibly transformative. It's going to reshape how we interact with our governments, how, you know, our economies work, how businesses work, how we do science, how we do education. And then the question is, if it's going to be such a transformative technology, who would you prefer as building that? United States, its European allies, China, Russia, India? My gut feeling is that it's a much better future for the world if technology that is going to be, sort of, a fundamental building block for the way in which the world operates, is aligned with Western liberal democratic values — things like the freedom of speech, as one key example.
Failing that, you would find the sort of West's ability to influence broader conversations in the tech space, I would say, significantly diminished. And I think it's actually something that China has sort of been betting on, is that while the U.S. might get to, let's say, I want to say AGI, but I mean basically, increasingly advanced AI-based systems. They might sort of lead in the development of this, but China is going to bet more on scaling it, being able to diffuse it throughout the ecosystem. They're working actively with their partners across Africa, Southeast Asia, building up digital infrastructure. And the idea is that, while the U.S. might have the sort of biggest, shiniest model, the rest of the world is going to be running on Chinese AI, if the U.S. isn't careful.
And then the point is, is that even if you have the sort of big, shiny AI object, nobody's using it, what’s the, you know, what's the benefit there? And we are seeing some of this, like, when it comes to DeepSeek, right? You know, one question that I like to ask is, what's scarier? The fact that China's having to create this model, that the fact that basically all of academia and many countries around the world dropped everything they were doing and ran straight to it, right? And I think that's something that policymakers really need to sit down and have a hard think about, which is that, if there's a country that can create a model and everybody's willing to just run straight to it and start innovating and building off of it, you probably want to be taking that a little bit more seriously and make sure that you have something to offer as well.
And as a, you know, a similar sort of note, kind of moving away from the U.S. and the China dimension, I hope policymakers in Europe are also sitting down and, you know, having a really solid think about the implications of this. They have more compute. Theoretically, they have a lot of talent, great universities. There's no reason the innovation from DeepSeek, you know, had to happen in China. It could have happened anywhere, but it didn't. And I think there are some really big implications for that as well, in terms of, you know, what does it mean for the future relevance of, for example, the European Union or other countries who, you know, do have access to compute, who do have access to talent as well, who do have access to capital, but they're not innovating at the state of the art.
Lennart: Building on top of Keegan’s points, yeah, I think I agree with all of it. And I think what we should also do here is, like, take a view which covers the whole tech stack to some degree, models being part of it. And for some reason I'm like, with models, I don't even think that that's sticky to some degree. Like, I think if Meta would come out tomorrow with a better model than DeepSeek, this would be quickly replacing a bunch of research, academia, like, other companies using it.
The thing which I'm more worried about is, like, the broader thing Keegan was alluding to is like, well, if they come in there in the country, build the whole infrastructure there, build the data centers there, build electricity there, all for 5G, this is more of the sticky architecture thing, and then the rest comes from it, right? Or you already get running our chips, you're already running our infrastructure? You're already running on a Huawei smartphone? Oh, here, here comes, here comes the model with it, right? So, we just really need to take, like, the whole tech stack approach here, and eventually trying to counter that, and having good, open-source models, or at least publicly available models, again, we should also differentiate these two things, might be a key advantage here. But again, like you need, like, a more holistic approach here, which I think is, like, totally missing right now. And we are all well aware of the Chinese Digital Silk Road, where they're trying to make such an offer.
One thing, which I would say, and like, connecting it to the previous point, is, like, if we look at the diffusion framework here, to some extent, a diffusion framework is trying also partially to solve that, there is a thing you can basically say, it's like, you guys are using our AI chips? Sorry, no more ties to these entities, no more Chinese models, for example. Again, this would be another blunt way of using it. It's technically fully legal and within the realms of export controls. And you could do so. I don't think one should do so. I think one should actually just like, have the better ecosystem, the better tech stack to offer, to think about it.
The question which I would actually pose to Keegan is like, how do you think this will go forward? Do you think just, like, the CCP will continue allowing all Chinese AI companies to, like, publish their open models? Because interestingly, what we see is actually, DeepSeek censoring mostly happens not on a per model level. There's like, something going on there, but most of the censoring is actually, like, on their deployment level, right? Like, it happens after the model has done this. It's like, do you think the CCP members will be like, worried about these kinds of things again, if like, the models are, like, less than certain to some degree, like, could also just undermine the regime in there? And now DeepSeek has all of the attention. Should we just expect it goes forward, never continue open sourcing and publishing these models?
Keegan. Look, my perspective on this is, people said the exact same thing about the internet, and that it would be like trying to nail Jell-O to the wall. You couldn't control it, and China wouldn't be able to do it, and we would see the fall of authoritarian regimes, the sort of spreading of democracy. And what we've seen is the exact opposite, with authoritarian countries actually having quite high Internet penetration rates, which have been able to effectively leverage control of the Internet to sort of improve their ability to surveil and control and grow in power the same way.
There's nothing that I see about AI that would suggest we're going to see something different. I think it's absolutely the case that China's going to be concerned about the sort of content that can be generated by AI-based systems. They do have pretty robust regulatory frameworks internally. They do have sort of standards in place to ensure sort of alignment with, let's say, party thought and whatnot. But the idea that they're going to walk away from AI while the U.S.is racing ahead, I just don't see that happening. And then, you know, the next question is like, okay, could they sort of survive with a closed source AI-based ecosystem, and I just don't see how that could happen when you see China committing to open source across the entirety of the tech stack. You know, they're huge supporters of Linux. They're making investments into sort of like risky architectures. They're working on developing open source operating systems for their mobile devices, for their desktops and laptops. They're basically across the entire tech stack, China's all in on open-source, and part of that is a response to sort of pressure from the U.S. and its allies to push them there.
The problem is, is that the U.S. didn't follow them into the open source competition spaces nearly as strongly as they should have, which allowed for Chinese influence to grow quite dramatically in that space, and I think that's quite scary. But now that people are slowly starting to wake up to the importance of competing in the open-source ecosystem, we might start to see a change. This isn't to say that open source is a cure all and that it's going to somehow fix everything in the world. My guess is, we end up at some sort of equilibrium where you have a substantial amount of closed source AI models that are perhaps more trusted. They're supported by sort of, you know, larger technology companies, which gives some air of, like, longevity and resilience and stability. You'll see open source models that are going to be quite competitive, which will probably, similar to open source software, be, you know, fairly important parts of the world's digital infrastructure. The only problem is that the U.S. was not competing nearly as much as it should have been in that space. We will see what changes as we go forward, but it's certainly an emerging area of competition, and basically all of China's AI stack at the moment is in the open source space, for example, Quentin as well. Yeah. I mean, Lauren, do you have something to add? Because I know you're quite big on this as well.
Brianna: Lauren, could you also walk us through a little bit some of the other side of the debate? Because there are reasons, right, why the U.S. hasn't followed China into the open source ecosystem. Walk us through some of the risks there and how we can find the right balance, in response to what Keegan just said?
Lauren: Yeah, so, I'm definitely an open source maximalist, but I do have tangible reasons for that. So, for the past over six months now, I've been working with Fortune 100 companies and CEOs there that are thinking about adopting third party AI systems or building them themselves. And I think there's this misconception that the bigger labs do have more trustworthy technology. And it turns out that when you're going through procurement on the enterprise side, it's actually kind of a black box to understand what's happening inside these companies and inside of these models, and we're in a bit of a situation where, you know, a lab presents a capability, or they show a demo, and we're in this scenario where the enterprise kind of really just has to trust that what they're saying is true. And we know that these models change and evolve, and that results aren't always the same, and, you know, the output may not be reliable, and things like this.
And so, we're in a situation now where companies are procuring systems from the big labs. Part of that is they think, you know, they're big. If something goes wrong, we could sue them. They have money, and if something goes wrong, we'll figure out how to recoup some of our investment. But the liability piece really isn't worked out yet around who ultimately pays if something goes wrong, and we're in this situation where it's, okay, trust me, we're big. We're a big company. We have a lot of investment. You should trust us. And what I'm hearing when I speak to some of these internal teams is they actually prefer open source, because they can pull the models into their own environment and do whatever tests they want. So they can probe, they can understand how they work, and that's not how things occur when you're testing closed source models.
And so, I would say that there's not only — it's fun to talk about kind of the geopolitical dimensions and the global dimensions of open source, but even when you zoom into one specific company that touches the lives of many Americans, or whatever it is that they operate, oftentimes, they do prefer open source. And we could talk about the safety dimensions of open source as well. I know people, you know, DeepSeek release their model weights, which is interesting and novel, and most companies don't do this. And there's a lot of talk about whether this is dangerous and enabling people to do dangerous things — nuclear risks, bioweapon risks, cyber-attacks, things like that. And also, it's this, it’s not all black and white, and things are moving quite quickly. And so, for me, the affordances of open source outweigh the risks of releasing model weights at this point in time, but it is important to track what's happening there. And as models improve, what capabilities are enabled by new advancements, and when you have these weights available, what is that kind of catalyze for the for the end user? So, I think it's important to track, so from a kind of like major existential risk perspective, there's a lot going on there.
And then, when I think about safety and more near-term and tangible risks, my view, which may be counterintuitive, is that I prefer having more people building, and so open source enables that, as we've seen. You know, researchers have rushed to leverage DeepSeek and so, the more people who have eyes on new technology and can come up with novel solutions that protect people from the potential harms of AI, I think the better. And I don't want those capabilities and those ideas centralized in a handful of labs or a handful of academic institutions. I want as many people thinking about potential solves as possible. And to me, that's what open source enables. And coming from big companies like Google and Meta, I've seen the centralization, both of problem identification and solution proposals, and I think that open source is the way to go here.
Brianna: It is really interesting, because, of course, right now, the U.S. and UK are spending significant resources on AI security and questions like, how do we protect model weights from cyber theft, particularly for more advanced systems, which might not be available now, but at some point in the future? Like, you know, with the report that Sella Nevo and his team put out at RAND, like, if we have more advanced AI systems and we need to protect them at a level four, level five, like, what does that look like, and what resource should be put to that? So, it is a really interesting debate that is going to change over time as the technology advances. And I think it's quite telling.
But, we've talked a lot about, some of the risks and, like, the national security implications at the global level, what about security risks to users, because there's also been some debate over the extent to which DeepSeek poses security risks to users by collecting personal information that could be shared with the Chinese government. For example, I've heard it been referred to as, like, the TikTok problem on steroids, but that's not exactly the whole story, right? Because the app will collect personal information if you register with your email address, but there's a lot of workarounds. You can download the model and ask it questions directly without having to go through the company processing the request.
Lauren, I wonder if you could just walk us through some of these other risks, like risks to individuals, the risks to users, the risks of censorship, misinformation. And then Lennart, I wonder if you can also walk us through some of the backdoor risks that you outlined in your in your excellent Just Security article today.
Lauren: I think it is really interesting that this conversation about DeepSeek is coming on the heels of the potential TikTok ban and so people and creators saying, I don't care if China has my data, if a Chinese is spy watching me, and they really didn't show — at least some of the creators that I saw on TikTok — didn't really show much regard for that. I think that considering DeepSeek, I imagine, touches a bit of a different community than TikTok creators. I am curious what the reception will be from users. I think there is more awareness that China does have access to your data.
But the nice thing about open source models is that you can deploy them on your own device and in your own environment, and so it doesn't necessarily have to be the case that you're sending data back to China. There are products that have come out, even in the past few weeks, where you can easily use Llama or other products that enable you to do this in your own environment, so that data exchange doesn't happen. I think there might be a digital literacy issue there that has to be addressed, but that's a potential solve there. And then, in terms of the impact on misinformation, censorship, you've seen, I mean, we've all seen the screenshots where people are asking about Tiananmen Square and then DeepSeek doesn't respond. And I think that this is — it just brings to light that users are more intelligent and aware, perhaps, than some people give them credit for. I think the fact that they know that this is a Chinese model, and they may be getting back information that isn't entirely accurate or reliable, is something that people are conscious of and publicizing. So, I'm not sure what the risk would be there in terms of just engaging with the model, but I'm sure there are other downstream consequences that haven't fully been considered.
Lennart: Yeah, I can say something about, like, vector risk or something, and that this becomes, like, more evident. Now, I think it's nothing new for everyone who's using a computer that, whatever you run on your computer, you should be careful. And there used to be a time on the Internet where people just like, you know, downloaded everything, installed it, and they had, like, another toolbar in the browser, even installing malware and whatever, and like, to slowly change over time, we went to, like, more authorized code. There's like, checking going on iPhones and Android being a prime example. You can actually only get apps via these app stores. That's being checked for.
The interesting thing about DeepSeek here is like, well, everybody's downloading it and everybody's running it, and I see two types of, basically, backdoors here, vulnerabilities here. One of them is, like, fairly traditional, just like, if you run code, and in this case, you run a gigabyte-sized file, there could be backdoors in there which allow remote code execution, right? We had vulnerabilities in PyTorch and other frameworks before, which allow you to deploy these models to run authorized code. So, that's just a thing to be very careful of. But it's the same with everything about open source, which is an ongoing discussion. If you just use a library on the internet, there could be vulnerabilities in there. Okay, not unique to open source. Could be any library. Security risks are there. There could be vulnerabilities in any kind of code you run, models not being an exception. The only thing is, it makes it bit harder to look for them because, again, this file is just a bunch of numbers, and you could hide things in there.
Then away from this traditional one is more like, well, we got this model, and it can be thinking, right? And what there is a nice paper from a leading U.S. company which talks about sleeper agents. So, what they were able to do is basically train the system in a way, if you gave it a specific trigger from writing good code, it started writing code with vectors, started writing malicious code. And I think that's the thing to be worried about. Like, these models can theoretically be trained under certain conditions, under certain code words, they change their persona. They change, like, they create malicious code, they maybe start saying different things. And that's really, really hard to check for, right? Like, you need to know what you're searching for there. And like, these models have, like, so much, again, in great knowledge being, like, gigabytes of files.
And I think that's the thing to be worried about, right? Like, if you run for the worst case scenario here, DeepSeek being the best model, or whatever's coming next, we integrate in all of our critical infrastructure, and then somebody just says the magic word, and suddenly all of these models don't work in the way we intend them to behave, or like, they start, for example, implementing back doors in code, right? Just for example, the model is being aware, oh, I'm being run within a leading U.S. tech company. Now I'm writing code with, like, back doors. There'd be a big security risk here, and that's just a thing to be tracking. And this will be really hard. This is also really hard, that I know Anthropic and Microsoft is not doing this to me, even harder for them, because I can't even check, but we have even the same issue for openly released models. And I don't see, like, an easy solution for this right now. We just need lots of testing, and the saver here is just like a transparent training process, and again, trust to these companies. And I think right now it's at least fair to say that I would trust OpenAI and Anthropic more to not have sleep agents in their models than companies which are putting out these models into the open.
Brianna: That seems like a huge vulnerability that just hasn't gotten sufficient attention, that policy makers are really going to have to tackle. Even then, there's no easy answer to it, as you say.
So, we've unpacked some of the myths and misconceptions surrounding DeepSeek when it comes to the importance of compute, the effectiveness of export controls. We've delved into some of the open source versus closed source debate. One thing that we haven't touched on yet, that I'd just like to briefly touch on if we have time, is about the energy piece of this, right? So, there's been a lot of posts floating around the internet where people are saying that because this model is more efficient, it's going to consume less energy, and isn't this a great thing. For example, one of the leading ethicists at the University of Oxford wrote on social media that this was her main takeaway from DeepSeek, that if American companies had constrained their energy consumption because it was the right thing to do, they might have gotten to a similar solution before DeepSeek.
And it just strikes me that this isn't quite right for a number of reasons. First, like, the comparison between DeepSeek energy consumption and American consumption is kind of misleading, because we know DeepSeek wouldn't have been possible without OpenAI’s model, for example. And second, you know, this idea that because DeepSeek is more efficient, we're going to have reduced energy costs overall, that also strikes me as being kind of overly simplistic, you know? So, maybe they've saved energy, they’ve saved energy in the training runs, but that could be offset by more intensive techniques for answering questions and, you know, producing longer answers. So, I've seen some initial figures floating around that, you know, one person testing performance of DeepSeek smaller models on a small number of prompts were suggesting that it could actually be more energy intensive when generating response than the equivalent size model from Meta. So, I think it was something like 87 percent more energy intensive in the end. So how do you respond to this, this idea that, you know, DeepSeek is going to be more energy efficient, and what does this mean in terms of energy consumption in the future of these models?
Keegan: To be honest, it just sounds ridiculous based on how you described it, for lack of a better word. And energy matters, okay, but China is light years ahead of the U.S., to be honest, when it comes to energy generation. I encourage people to look at the graph of how much energy China is actually creating. So, arguing that energy was a constraint somehow, to be honest, doesn't make sense to me from a starting point anyway.
I think energy is going to matter, absolutely. The U.S. grid and with the investments we're planning is not ready at all, to be honest, for the amount of compute that we're trying to build at the moment. That will change quickly. But the flip side of this is also that the U.S. is making huge investments into renewables and other sources of energy development, because, turns out, when it comes to companies who are building, you know, AI to cutting edge, they don't like to spend ridiculous amounts of money on energy. It's actually a pretty nice thing to have systems that run efficiently and with less environmental impact, because it means your costs go down, which is how you end up with companies running around, opening up retired nuclear power plants, looking at many reactors, talking about fusion. You know, the investments pouring into the sort of energy side of this are massive at the moment. If anything, the more work you're doing in AI, the better it is for energy demand in the long run, to be honest.
I'm not sure if there's been anything that has been as big of a driver to sort of improve how we're generating and using energy, you know, than what we've seen the last couple of years surrounding the build out of increasingly sort of large AI-based data centers. I mean, Lennart or Lauren might have more on that. But from my side, I just, I honestly don't understand that argument at all, really.
Lennart: Yeah, I can also just confirm. I don't really follow this reasoning here. Funnily enough, we just published, actually, a Rand research report where we talk about the exponential energy need for AI growth. So, like happy to point to this if people want to know more. And turns out, it's my favorite topic. It's another line going up on a log chart. We talk about an exponential increase here. And totally fair, this should raise, like, climate concerns, environmental concerns and other things.
But like, that DeepSeek now says, like, oh, we like, we're good, like, all this energy growth, the Stargate 500 billion thing, activating Fremont Island, has all been wrong. It’s mistaken, and I would just point to the whole conversation we just had about the importance of compute, and replace the word compute and replace the word compute with energy, right? When we say we need more compute for making the model think longer. When we say we need more compute to serve more users, when we say we need more compute to drive this economy impact, all of this equals more energy. It's true that, like, you get more compute for less energy over time. That's Moore’s law. That's what we've seen. But again, the trend in how much more compute we have is outpacing all energy efficiency trends here. So, the energy demand will be continue going up, and this will be a key — and is a key — policy topic in the U.S., even in U.K. right now, where they're talking about, like, these special zones to build these data centers to make the permitting easier here. So yeah, these are my takes. Then again, I would refer to my paper to talk more about these things.
Lauren: I agree with all of these points, and I think it's important to keep an eye on what's happening in the broader, more mainstream dialogue around energy. The point you made, the point this ethicist put forward, I think, is quite illuminating for how people think about what is happening with AI and energy consumption. I know water is a big topic, and so, it's a way to create this binary of all good and all bad. And so, if AI uses all this energy, it's terrible. If it doesn't, it's better. And so, I think that that's a conversation that continuously needs to be recalibrated as more information and data emerges, and I'm very excited about the market opportunities that are emerging, like financialization of energy consumption and things like this. And people are innovating to develop clean energy and do it in a way that is more sustainable moving forward.
Brianna Rosen: Yeah, exactly my perspective as well. I just didn't understand the argument, but I think it just points to the fact that there are so many misconceptions surrounding DeepSeek, like the fact that it's more efficient on the compute side means less energy consumption, and therefore somehow more ethical than what American or European companies have done, just shows that I think people that are talking about the ethics of this and the environmental risks of this really need to dig deeper into the technology itself to understand where this is going and what this means.
Well, we're almost at time here. So, I just want to ask each of you if you can reflect really briefly about, so, given all of the things that we've talked about, about some of these misconceptions surrounding DeepSeek in the popular domain, is DeepSeek really a Sputnik moment for AI? I think I know some of your answers based on our conversation, but would like you to each briefly reflect on that by way of closing, and then where do we go from here? So, what does this signal in terms of what we can expect to see five to 10 years out, realizing that AI development, of course, is neither linear — it doesn't progress in a linear line, but it's difficult to predict. But where do you see this heading in the next five to 10 years?
Lauren: That's quite a big question. I mean in terms of AI, I think this, not that it was necessarily a Sputnik moment, but just that this is a global flashpoint for AI development and diffusion. And I think most people hadn't really been paying attention, it seems. And so, maybe for us that are in the industry and see this day to day, it kind of followed along specific trend lines. And maybe it was interesting that it came from China and not another country, and that kind of changes how we view things and what our work might look like moving forward, but I think it really was a moment for people outside of the industry to wake up, and I don't know — that my concern is that they don't necessarily know what it means, like what follows from here, and what should my, as an individual, what should my takeaways be?
But for me, as someone who works in AI and AI governance, it's only that AI diffusion will continue the technological capabilities, and the advancements are continuing at a rapid pace. The reliability of agents is incredibly important to me, because if we want AI to be used in our day to day lives and critical industries, they need to be reliable, trustworthy and safe. And so, I think this changes the conversation a bit and provides more opportunities for helping to facilitate that.
Brianna: Lennart or Kegan, either of you want to jump in?
Lennart: Sure, happy to. Yeah, I wouldn't call it a Sputnik moment. I think it definitely caught me by surprise as much it caught others by surprise. So, you know, we should all like, I think, yeah, it's always nice — it's like, wait, we said this a year ago. It's not enough. You know, Keegan wrote about open source half a year ago, but it turns out now's the time to push it, right? Now, we have, like, this public attention, talk about it. It just really hit home, like, a couple of sweet spots: U.S.-China tech competition, all of it during Trump's first week; it hits on open source models versus closed model weights; it talks about export controls, which has been a topic. All of these are important topics. All of these have different policy implications. All of these things need to be thought through.
The thing which I would just not forget here is, like, couple of months ago, people are saying, oh, we hit the limits of AI, like, well, doesn't really look like it right? Like the line continues going up. AI capabilities continue to improve. And I think this should, like, be a broader message here. I'm glad everybody's freaking out about all of these different topics here. I think we should more broadly think about, like, increasing our capabilities and what it means. OpenAI just release deep research, and again, if researchers are listening to this, give it a try and compare it to your research assistants. I think they have some actual competition right now, and these things will have economic impacts here. So, going forward, in a couple of years, we need to think about all of these topics. We will need to continue iterating and improving export controls and be more agile about them. We need to think about the open and public debate. And I think we had a great discussion here, and I think it will be really hard to strike the right balance here, but we also need, in general, like, try to manage increasing AI capabilities and see how they will impact our society and our world, and then how they will diffuse around the world.
Could easily be the case — again, you ask about 10 years out there, I generally only talk about the next year with AI, but if you look 10 years out there, I think we could easily imagine a story, like, how we missed the boat on diffusing AI technology and China built out the digital Silk Road here, right? So, we got the diffusion framework right now, which is a nice protect move, but we need, like, more of these promote moves, actually get the tech out there, build these ecosystems. And only building the best models won't be enough. Build infrastructure, give out the smartphones, give out 5G, all of these other things. Otherwise you might be looking back at, again, 10 years from now, and we lost AI ecosystem, not because we don't have the best models, because, actually, we didn't get it out there. We didn't give it to the people. And again, we need to do all of this while managing the security risk. And I think this will be a fundamental challenge.
Keegan: I think from my side, also definitely not a Sputnik moment. If anything, it actually just reaffirms American dominance in the field. We saw that compute was a barrier. We saw they had to react to that. We did see that DeepSeek had problems scaling this when it got international attention. However, it also shows, don't underestimate China. They have made the strategic priority. They're pouring huge amounts of resources into this. They want to be the world leader. They are building out the world's infrastructure to be able to run AI-based systems. Yes, they can do that, to be honest, with their domestic chips at the inference level, as the production capabilities start rolling out, and that's something that we need to be prepared for.
It was only a couple of months ago we had senior lawmakers in the U.S. saying that the PLA’s use of an outdated open source Llama model was a national security threat. And then a couple of months later, we're talking about the new Sputnik moment, how out of the blue, China is somehow, you know, light years ahead of us. That's, you know, that's not true, but the U.S. has made a mistake. Not everyone, but a number of folks, have made a mistake in underestimating China and their capabilities. And we have seen that China is incredibly talented and is capable of innovating across the entirety of the sort of emerging technological infrastructure, not just AI, but I'm thinking 5G, quantum, IoT, solar, et cetera.
Now what this tells me, and kind of agreeing with some of the things that Lauren and Lennart have been saying, is that the U.S. definitely has the protect side down. I think the U.S. understands the necessity of export controls related to compute. You know, this will help build a mode, but where there's still substantial opportunity to do more is on the promote and the innovation side. So, how do you encourage more innovation domestically? How do you encourage, you know, the development of a broader open source AI ecosystem? How do you create the sort of ecosystem that will encourage, you know, more development, more innovation. But also, on the promote side, how do we make sure that the rest of the world is coming to the United States to work with us on building out what, to be honest, is going to be the future global digital infrastructure? What does it mean for the United States be able to make sure that its AI-based solutions are used across Europe, Africa, Southeast Asia, South America and so on, and not China's?
So, looking forward Sputnik moment, not so much. Think the U.S.is still comfortably in the lead. Yes, they should take it seriously. But to maintain that lead, in addition to the protect, they have to take to promote the innovation part seriously as well.
Brianna: Well, thank you all so much, Keegan and Lennart and Lauren. This was a really good conversation. I'm glad that we had an opportunity to dig into it a little bit after some of the hype has passed and people had time to think through what's going on.
Keegan: Thanks for having me.
Lennart: Thanks for having me.
Lauren: Thank you. This was great.
Brianna: Thank you all for those insights in the rich discussion. DeepSeek’s emergence has certainly captured global attention, particularly in Washington, DC. But as you've all underscored, it's hardly the AI apocalypse, and as we've discussed, the U.S. maintains a leading position in many key areas of AI development and deployment, and it's well poised to stay ahead of the global AI race, provided policy makers and industry leaders continue to adapt. We'll be watching closely as the debate on open source AI continues to unfold and tracking whether this moment truly reshapes the global balance of power when it comes to AI, or simply drives more investment and innovation within existing ecosystems.
That's all for this episode of the Just Security podcast. I'm your host, Brianna Rosen, and this episode was produced with help from Paras Shah and Clara Apt. A huge thank you to our guests, Keegan McBride, Lauren Wagner and Lennart Heim, for generously sharing their expertise and insights. If you enjoyed this conversation, please leave us a five-star rating on Apple podcast or wherever you listen, and be sure to visit our website, justsecurity.org, for more in depth coverage of AI and emerging technologies. We'll be back soon with another deep dive into tech policy. Until then, thanks for tuning in.